By Kayla Downs
How does Google suggest searches? AI. How about when Facebook recommends people to tag as soon as you upload a picture? Yep, that’s AI facial recognition. The thousands of flights that occur every day? They are mostly being flown with AI. While many people fear AI is going to take over the world, it is already present in most parts of our lives. Artificial Intelligence (AI) is “the ability of a digital computer or computer-controlled robot to perform tasks commonly associated with intelligent beings” according to the Encyclopedia Britannica (Copeland, 2019). AI has been getting huge attention this past year and is being employed in new fields everyday.
Environmental science, specifically field testing, was long thought to be a place where AI couldn’t be as accurate or successful as humans. AI can be used to make graphs, and predict trends overtime, but the actual data must be collected by humans. Chinese scientists published a paper this year detailing how AI was used to identify characteristics of water pollutants and track pollution sources. Their AI used long short-term memory (LSTM) with cross-correlation to identify the point sources of water pollutants (Wang et al. 2019). LSTM refers to the property of AI models by how they train them. For example, think about training an algorithm to recognize cats. You would show it a bunch of pictures of cats and it would find common attributes they all have in common, like fur. But, you don’t want the machine to think that everything that has fur is a cat, that’s not true. Developers fine tune the machines so it can remember that cats have fur long enough to recognize other cats, but not for so long that it believes everything that has fur is a cat. This long-and-short type of memory is taught to the algorithm and allows the algorithm to correctly identify water pollutant types and locations.
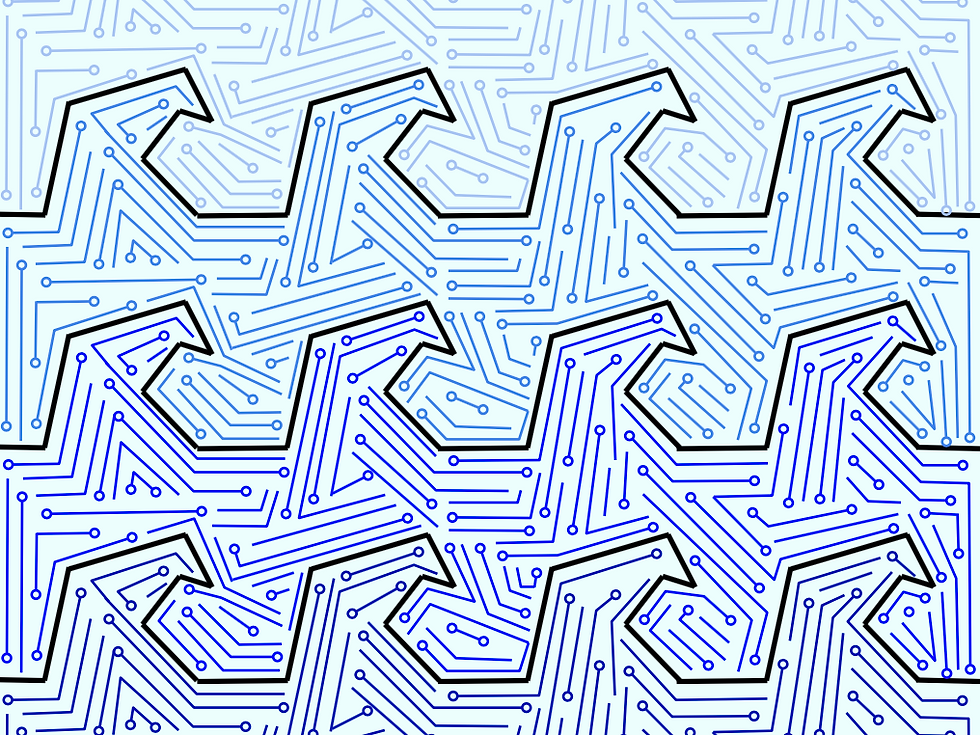
Point sources are specific geographical areas where water pollutants can leach into river basins (NOAA, 2017). Common water pollutants, and those identified in this study, include dissolved oxygen, nitrogen, phosphorus, and metals like mercury, lead, and arsenic (Wang et. al. 2019). For the algorithms that run AI machines, they are trained using data that the researchers know is correct. Water pollutant data that were collected in the past are used to train the algorithm, and the results verified based on subsequent observations. The property of AI to behave like a human brain and learn flexibly is where the contradictory long short-term memory comes in. LSTM allows the algorithm to remember attributes but also adjust what it has learned, just like humans can do when presented with new information (Mittal, 2019). The researchers in the water quality project utilized LSTM and presented it with data of water pollutants (what they are and where they come from). To make is as accurate as possible, data sets were cross-correlated with each other. Cross correlation can identify abnormal fluctuations in water pollutants quantities.
This AI scheme accurately and quickly identified pollutants and the industries discharging pollutants. These pollutants can seriously harm water quality and having this information allows better management and control of pollutants in river basins in the future.
Algorithms like this one are transforming data collection and interpretation because they can recognize patterns in data faster and more accurately than a human brain would be able to. They can conquer huge amounts of data in seconds, allowing scientists to spend less time aggregating the data and more time understanding what the patterns mean. AI changed the world before 2019, but it is constantly evolving and being integrated into new industries at an increasingly rapid pace, as seen in projects like this one.
References:
Copeland, B. J. (2019). Artificial intelligence. In The Encyclopedia Britannica. Retrieved from https://www.britannica.com/technology/artificial-intelligence
Mittal, A. (2019). Understanding RNN and LSTM. Towards Data Science, Retrieved from https://towardsdatascience.com/understanding-rnn-and-lstm-f7cdf6dfc14e
Categories of Pollution: Point Source. (2017). NOAA Ocean Service Education. Retrieved from https://oceanservice.noaa.gov/education/kits/pollution/03pointsource.html
Wang, P., Yao, J., Wang, G., Hao, F., Shrestha, S., Xue, B., … Peng, Y. (2019). Exploring the application of artificial intelligence technology for identification of water pollution characteristics and tracing the source of water quality pollutants. Science of The Total Environment, 693, 133440. https://doi.org/10.1016/j.scitotenv.2019.07.246
コメント